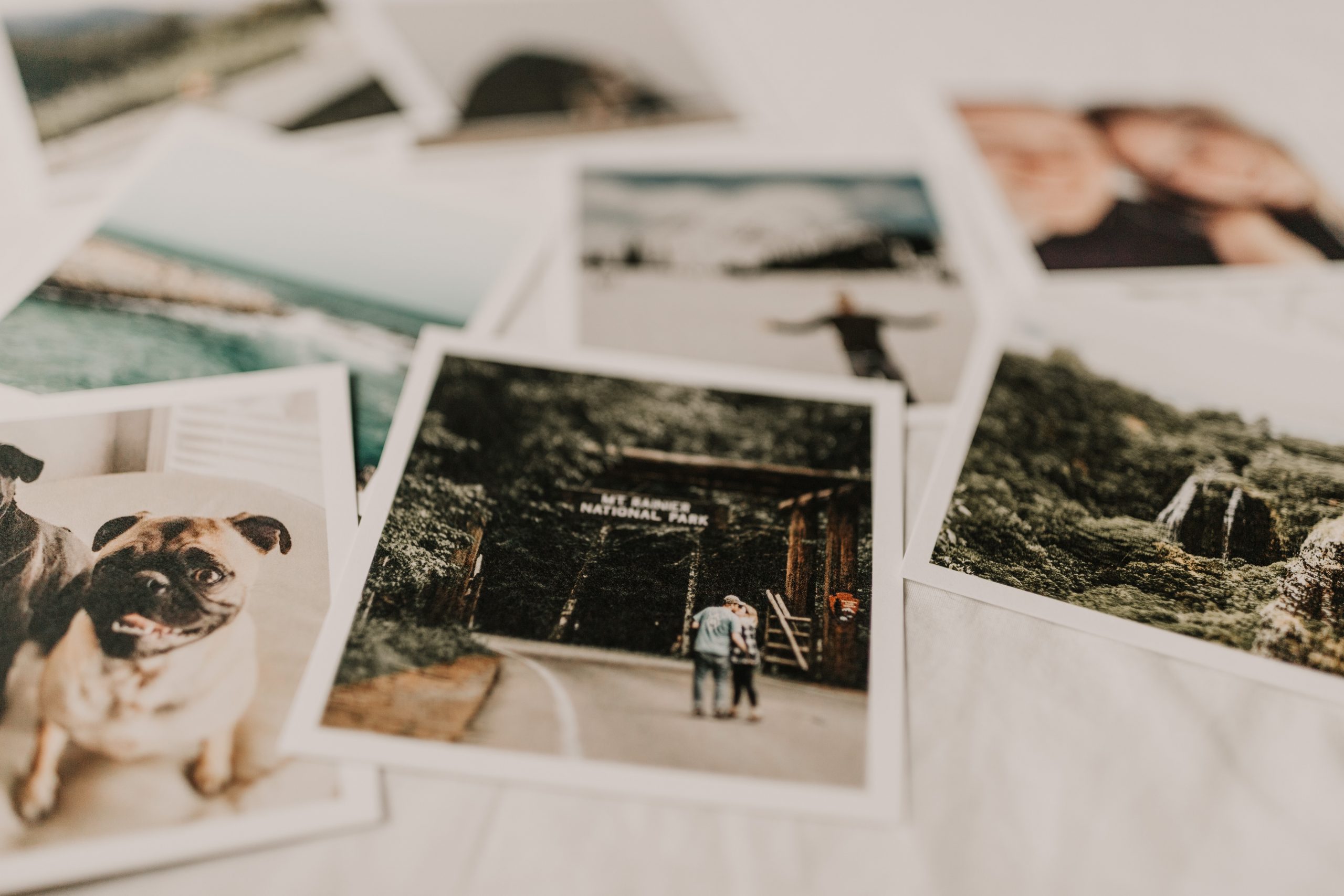
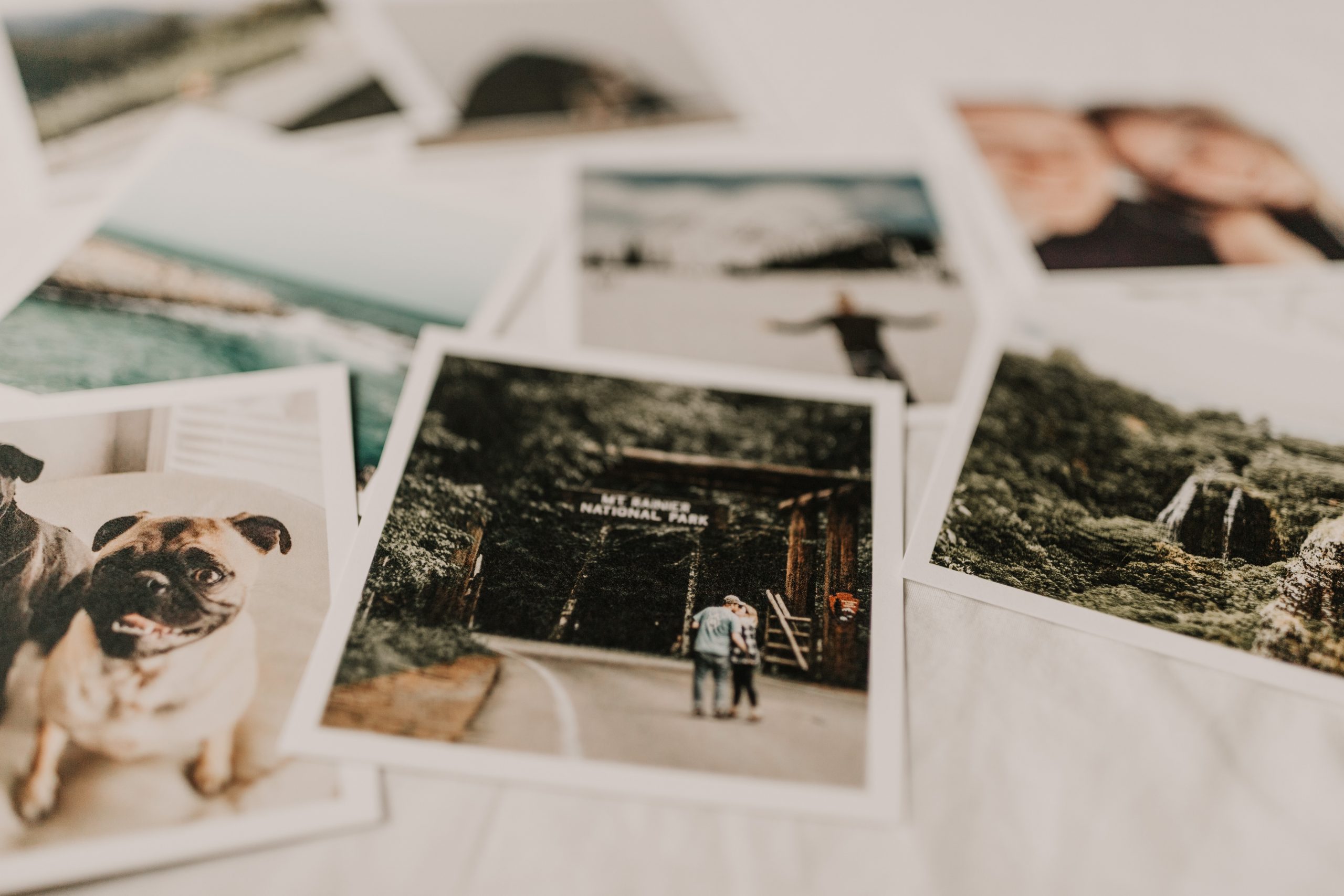
Automating the alternative descriptions of images
While collaborating with a school publisher for a textbook pilot project, we wondered if it was possible to simplify (and possibly automate) the image description process. From this question, we worked at a pilot project – presented for the first time at the 2019 Digital Publishing Summit in Paris – on the possibility of automatically generating alternative descriptions of images through the use of artificial intelligence.
The big technological operators (Microsoft, Google, Amazon, Facebook) already offer services based on artificial neural networks and machine learning to add an automatic description of the photographs posted by users on their platforms. We therefore asked ourselves if it was possible to use AI to automate the alternative description of images in the publishing world as well.
Due to the complexity of the images in the books, the normal solutions currently available on the market are not enough. Starting from these considerations, as LIA we have started a research project to test the use of some AI algorithms already available on the market now applied to digital publications.
The phases of the project
Before starting it was necessary to define a template for the creation of alternative descriptions, consisting of two complementary parts:
- image category, a taxonomy of categories to classify the different types of images (for example: art, comic, drawing, logo, photograph, etc.);
- image description, image description, that is the actual description of the content of the figure.
Once the services have been chosen among the existing ones and an algorithm has been trained, we developed a tool that receives an EPUB file as input, extracts all the images present within it and automatically creates the alternative description, consisting of its two elements (category and description). Some types of images have been excluded, such as comics, graphics, maps and signatures, of which the outputs obtained from the tested services are totally random.
At this point, as a final phase, it was possible to test the prototype on some files provided by publishers, obtaining the following results:
- image category automatically generated: 42% accuracy
- image description automatically generated: 50% accuracy
Next developments
Thanks to the work carried out during the pilot project, we were able to see first of all that the image recognition algorithms currently available on the market have been optimized for photographs, which are more present on the web, while they are not able to describe other images (such as drawings, works of art, logos, graphics and infographics), more present in publications of all kinds.
We think that the accuracy of the image category can be improved by refining the initial training dataset of the service used, while for the description it is still necessary to wait for an evolution of the algorithms available on the market. However, considering the speed with which technology advances today, we plan new tests over the next two years to check for any improvements in automation.
Alongside the research on this issue, we always work to create more awareness in the publishing world on the importance of accurate alternative descriptions. We do this through training in specific meetings aimed at professionals in the sector: editors, editorial editors, graphic designers and illustrators.
Not only that: we offer a consulting service to companies and publishing houses specifically aimed at writing alternative descriptions that are punctual and suitable for the context in which they are found.